Primena tehnika vizualizacije u bazičnoj statistici.
Dejan Pajić. Univerzitet u Novom Sadu, Filozofski fakultet. 2020, – 283 str. ISBN 978-86-6065-582-2
Besplatna, PDF verzija
i interaktivna verzija knjige.
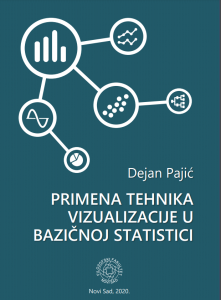
Ovaj interaktivni udžbenik iz oblasti bazične statistike (kao i pdf verzija), po rečima autora „napisan je za potrebe kursa Uvod u statistiku na osnovnim studijama psihologije na Filozofskom fakultetu u Novom Sadu, ali je namenjen svima koji žele da se upoznaju sa ključnim principima statističkog rasuđivanja i osnovnim postupcima statističke obrade podataka. Većina odeljaka u udžbeniku propraćena je odgovarajućom interaktivnom vežbom koja podrazumeva da čitalac bude aktivno angažovan u kreiranju podataka i njihovoj interpetaciji uz pomoć različitih tehnika grafičkog predstavljanja. Udžbenik je dostupan i u PDF verziji, ali u njoj nije moguće koristiti napredne opcije koje su podržane u veb verziji.
Odeljcima udžbenika pristupa se sa stranice Sadržaj. Preporučujemo da se pre prvog čitanja udžbenika upoznate sa njegovom strukturom, a potom krenete od poglavlja Uvod. U gornjem desnom uglu svake stranice nalaze se linkovi za navigaciju pomoću kojih možete da otvorite različite stranice, pređete na naredni odeljak udžbenika ili da se vratite na prethodni. Kada postavite pokazivač miša preko ikonice prikazuje se umanjeni sadržaj udžbenika pomoću koga lako možete da utvrdite na kojoj stranici se trenutno nalazite i da pređete na neki drugi odeljak. Kliknite istu ikonicu da biste videli sadržaj u punoj formi.
Pored interaktivnih vežbi, u nekim odeljcima postoje i pitanja na osnovu kojih možete da procenite u kojoj meri ste razumeli i savladali upravo pročitani tekst. Kliknite na pitanje da biste prikazali okvir sa odgovorom.
Otvaranje interaktivnih okvira
Većina odeljaka u udžbeniku sadrži interaktivnu vežbu koja se prikazuje u zasebnom okviru na vrhu ekrana. Okviri se otvaraju na dva načina. Prvi je klikom na odgovarajuće linkove u tekstu koji su vizuelno drugačiji od ostalih linkova, a drugi je klikom na link (strelicu) u vrhu ekrana. Strelicu u vrhu ekrana možete da upotrebite za zatvaranje okvira i za njegovo ponovno otvaranje. Klikom na strelicu otvara se okvir predviđen za poglavlje, odnosno deo teksta koji trenutno čitate, što znači da se na različitim delovima iste stranice mogu otvoriti različiti okviri.
Pretraga udžbenika
Na stranici Pretraga nalazi se okvir za pretraživanje udžbenika. Kada u okvir unesete jednu ili više reči i kliknete taster , biće prikazana lista delova teksta u kojima se pojavljuje traženi izraz. Prikazani delovi teksta su ujedno i linkovi ka odgovarajućim stranicama udžbenika. Prilikom unosa termina za pretragu možete da koristite simbol * (zvezdica) kao džoker kojim se zamenjuje bilo koja niska slova. Na primer, izraz stat* test* možete da upotrebite da biste pronašli delove teksta u kojima se javljaju fraze statističko testiranje, statistički testovi, statističkih testova i sl.
Registracija korisnika
Udžbenik je dostupan u režimu otvorenog pristupa i distribuira se pod licencom Creative Commons license Attribution-NonCommercial-ShareAlike – CC BY-NC-SA. Detaljno objašnjenje značenja CC licenci možete pronaći na Nacionalnom portalu otvorene nauke. Registracija i prijavljivanje nisu obavezni, ali su neophodni da bi se koristile napredne opcije. Napredne opcije podrazumevaju podvlačenje, postavljanje obeleživača i beleženje. Podaci koje ostavite prilikom registracije neće biti deljeni trećim licima, niti ćete na navedenu imejl adresu dobijati poruke, osim u postupku registracije i verifikacije. Da biste se registrovali, popunite kratak formular na stranici Prijava. Nakon prijavljivanja, na traci za navigaciju sa desne strane okvira sa tekstom pojavljuju se dodatni linkovi za podvlačenje, obeležavanje i dodavanje beležaka.
Podvlačenje
Delove teksta možete da podvučete tako što kliknete taster i prevučete pokazivač miša preko teksta. Nakon toga, podvučeni delovi biće izlistani na stranici Moj udžbenik koja postaje dostupna nakon prijave. Kada je neko poglavlje otvoreno, možete da se pozicionirate na podvučene delove teksta pritiskanjem tastera W (naredni) ili Q (prethodni). Da biste uklonili podvlačenje, kliknite taster i potom podvučeni deo teksta. Sve dok je taster pritisnut, možete da podvlačite različite delove teksta. Da biste izašli iz režima podvlačenja, kliknite ponovo taster na traci za navigaciju ili taster Esc na tastaturi.
Obeležavanje
Dodavanje obeleživača je slično podvlačenju, ali služi za jednokratno označavanje dela udžbenika na koji želite da se vratite u narednom pristupu. Deo teksta možete da obeležite tako što kliknete taster i prevučete pokazivač miša preko teksta. Nakon toga, odabrani deo teksta će se videti na stranici Moj udžbenik i služiće kao link do mesta na kojem je postavljen obeleživač. Da biste uklonili obeleživač, kliknite taster i potom obeleženi deo teksta. Kada obeležite neki deo teksta, prethodni obeleživač se automatski briše.
Beleženje
Beleške možete da dodate tako što kliknete taster i potom željeni deo teksta. U otvoreno polje unesite belešku i kliknite taster . Sve unete beleške izlistavaju se na stranici Moj udžbenik. Kada je neko poglavlje otvoreno, možete da se pozicionirate na beleške pritiskanjem tastera S (naredna) ili A (prethodna). Da biste prikazali belešku, postavite pokazivač miša na ikonicu . Da biste uklonili belešku, kliknite ikonicu i potom taster u gornjem desnom uglu okvira za unos beleške.
Tehničke karakteristike
Za izradu elektronskog udžbenika korišćeni su programski jezici HTML, Javaskript i CSS koje podržavaju svi veb-pregledači. Međutim, preporučuje se upotreba pregledača Chrome, Firefox, Edge ili Safari. Takođe se preporučuje ažuriranje odabranog pregledača, odnosno instaliranje njegove najnovije verzije. Tekst na stranicama prilagođen je za prikazivanje na mobilnim uređajima, ali zbog specifičnosti interaktivnih delova udžbenika, poželjno je da širina ekrana bude najmanje 1.000 piksela (px). Na užim ekranima moraćete da pomerate (skrolujete) interaktivne delove po vodoravnoj liniji kako bi svi elementi bili vidljivi.
Sve vizualizacije u udžbeniku izrađene su uz pomoć Javaskript biblioteke D3 – Data-Driven Documents koja se distribuira u skladu sa 3-Clause BSD licencom. Autor biblioteke je američki programer i stručnjak za vizuelizaciju podataka Majkl Bostok.
Većina primenjenih algoritama za statističku obradu delo su autora udžbenika. Složeniji postupci obrade podataka obavljaju se uz pomoć besplatne Javascript biblioteke jStat. Za ispisivanje formula upotrebljena je besplatna Javascript biblioteka MathJax.“
… za svaku preporuku.
Pročitajte više