Artificial Intelligence: Will it be fair, unbiased and non-discriminatory?
A panel discussion on ethics in AI research and development
Can AI be better than humans?
U martu 2019. u Montrealu je održana panel diskusija na temu etike u domenu istraživanja i razvoja veštačke inteligencije Artificial Intelligence (AI): Artificial Intelligence: Will it be fair, unbiased and non-discriminatory? Can AI be better than humans?
Prema navodima organizatora: Montreal is cementing its position as the hub for research, development and innovation in the field of Artificial Intelligence (AI).
Kao autor ovog bloga, postavio sam sebe u ulogu četvrtog (virtuelnog paneliste – DV). Moji doprinos diskusiji je priložen na srpskom jeziku, dok su odgovori stvarnih panelista preneseni u izvornom obliku.
U uvodu teksta navodi se:
This is a source of pride; however, a significant segment of our community is concerned about ethical issues in AI and how biases and prejudices may inadvertently be fed into machine learning algorithms because of the skewed historical data which they may be learning from. In today’s high-tech age, we are also trying to evolve into a more accepting, diverse and inclusive society where each individual has an equal opportunity to succeed irrespective of their background, ethnicity, gender or sexual orientation. If historical data used for AI research is not representative of the population at large, then AI may end up perpetuating human behaviour of discrimination towards minorities.
DV “… because of the skewed historical data which they may be learning from… If historical data used for AI research is not representative of the population at large“.
Ovde već izlaziimo iz polja statističkog i ulazimo u domensko znanje. Naime, možemo govoriti o objektivnim i subjektivnim greškama u podacima. Tako, istorijski podaci vezani za određene kategorije mogu biti objektivno čisti u statističkom smislu (očišćeni od ekstremnih vrednosti i različitih tipova grešaka, rešeni nedostojaćih vrednosti i sl.) ali dalje mogu u sebi da nose u subjektivnu iskrivljenost (pristrasnot) usled evolucije u značenju tih podataka. Npr. ukoliko idemo dovoljno daleko u istorijske podatke suočićemo se sa značajnim razlikama u stavovima opšte populacije po pitanju tzv. „marginalizovanih grupa“, posebno LGBTQ+, (o Romima da i ne govorimo, njihov status je i dalje van svakih razumnih ljudskih, pa i statističkih, okvira).
Domenski eksperti – (Data science translators, Behavioral psychologist i Data storyteller) moraju da zauzmu svoje mesto u AI (data science) timovima. Oni su ljudski eksperti, zaduženi elimimisanje subjektivnosti u podacima, rezultatima i naročito u njihovom tumačenju.
I sama naučna metodologija mora biti podložna promenama. Samo je pitanje vremena kada ćemo uvesti novu varijablu u set tzv. demografskih pitanja: Pripadnost populacij:i 1. ljudi 2. mašine. Trebalo bi što pre, pošto mašine (roboti, računari) već donose neke, čak i veoma važne odluke (u oblasti HR, recimo). U nastavku teksta, u svojim komentarima, pridržavaću se ove klasifikacije.
This is indeed a huge cause of concern and to address these issues, Queer Tech MTL, in collaboration with Montreal AI ethics institute, hosted a panel discussion on the topic, “AI: Where do we fit in?”, at the Montreal headquarters of Element AI, Canada’s largest homegrown AI company.
Despite a major snowstorm that day, Element AI witnessed a gathering of easily over a 100 LGBTQ+ tech professionals eager to learn more about what researchers are doing to address all these burning issues and also to give their feedback on how to incorporate ethical principles into machine learning algorithms. The event kick-started with networking over beer on tap accompanied by pizza (a regular feature at all QueerTech events). As the attendees warmed up inside the swanky high-tech offices of Element AI, Alexandre Vincart-Emard, CTO from Enkidoo Technologies who moderated the panel for the evening took centre stage to stump the panel with his well-prepared questions.
The three panel members for this extremely crucial discussion are experts in their respective fields and at the forefront of AI research; Audrey Durand (AD), Abhishek Gupta (AG) and Martine Bertrand (MB) had to rack their brains hard for about an hour to comprehensively answer the various challenging questions posed by Alex and the audience. You could witness the energy exuded by the attendees and these snippets of our conversation provide an overview of the evening.
To start off the discussion, the panel was asked to present their opinions about ethical issues and fairness related to AI, especially in terms of the notion of fairness, which is changing with time as society evolves; concepts of fairness can also be relative to regions or cultures.
AG: Responsible AI is the need of the hour and certainly a process needs to be established for the same, but how do we define a standard process? There’s no one single body which can do that because it won’t be representative of the whole population; hence, we need to leverage insights from the community and involve people who are actually going be affected by these decisions.
AD: Machine learning algorithms will never be fair and ethical when deployed in different countries and regions unless the same definition is agreed upon, or adapted versions come into the picture. Unfortunately, critics generally base their perspectives on how they want society to be, but we first need to overcome our own biases before we expect AI to reach that level.
MB: We as humans are striving to set boundaries for machine-learning models but it is going to be very difficult because AI will still reflect the biases and prejudices fed to it because it’s impossible for human beings to not have unconscious biases. So, this is still a very grey area.
DV: Ne može populacija mašina da bude bolja od ljudske populacije koja ih je programirala. Tek kad se uspostave ljudski standardi –(među ljudima) možemo govoriti o pravičnosti mašina – baziranim na AI. Naravno, standardi se mogu uvoditi postepeno, u određenim oblastima, čim se budu definisali.
Could you offer examples of governments and organizations that are making efforts to be accountable regarding the topich of AI ethics?
AG: Canada, with its progressive policies on diversity and inclusion, is certainly at the forefront — tremendous work is happening on principles governing AI internally within the government.
AD: AI Research labs are concerned about ethics and are incorporating feedback from communities to address how fairness needs to be handled by a variety of algorithms. For example, committees have been established in healthcare to ensure standard protocols for all types of patients.
MB: Most public institutions are making efforts to address fairness otherwise it becomes difficult to establish partnerships with stakeholders involved.
DV: Voleo bih da vidim tu pravičnost među ljudima. Nisam siguran u iskrenost vlada i organizacija, više se hvale nego što se mogu podičiti rezultatima. Svakako treba pitati javnost šta misli o tome.
AI is kind of like a ‘black box’, being dependant on the data it derives its insights from. Thus, there can be a trade-off between accuracy and fairness when it comes to judgement or decisions. Should there be regulations governing this and how can they be implemented?
AG: By working with machines rather than working against them. We must highlight the complementary points between humans and machines that we can leverage from. As AI evolves over time, its behaviour may change and the outside parameters set initially may no longer be adhered to, thus requiring human intervention. Constant monitoring will be required to reinforce the systems.
MB: What scares me the most about this is that we may project our own insecurities on the machines. And since humans are lazy, we may just start relying on solutions presented by AI, which can have dire consequences. Hence, we must not defer from exercising our own judgment as humans.
DV: Uzalud je računati na kompromise kada je reč o mašinskom učenju. Kao i kod svakog drugog „black box“ rešenja i ovde važi GIGO (garbage in, garbage out) koncept. Lično, smatram da jedna fina nota „ljudskosti“ u donošenju odluka (presuda) treba da postoji ali je pitanje kako uspostaviti merljive skale vrednosti ove karakteristike.
AI nikad neće imati emocije i intuiciju. I ne mora. Treba da ostane nešto po čemu će se veštačka i ljudska populacija razlikovati.
What are your thoughts on the controversial ‘GAYS’ algorithm tested by Stanford University recently, which identified homosexuals based on certain facial features with 91% accuracy? Something like this can surely have disastrous consequences on our society’s basic foundations which we are trying to establish.
AG: That algorithm is absolutely absurd. I spoke to the people undertaking the research and learnt that essentially what they did was to identify people’s sexual orientation based on facial hair and the angle at which people were taking their pictures. This is the prime example of how certain research can go horribly wrong especially if you don’t take into account the ramifications it can have on the society at large.
DV: Čemu opšte ovakva istraživanja služe, sem da zbunjuju ljude, koji su ionako konfuzni usled poplave raznih populističkih tekstova na temu AI? Trudiću se da lično ne budem pristrasan i ne smatram ovaj eksperiment teorijom zavere i čudi me da se Univerzitet Stanford upustio u ovu pustolovinu – naročito što je publikovao dobijene rezultate. Lično smatram da je ovaj “eksperiment” etički, koliko i kloniranja živih bića. Sem toga, u statističkom smislu 91% tačnosti nije neki naročito dobar rezultat (običajena granica nivoa poverenja se uspostavlja na 95% ili 99%).
Let’s touch on the topic of bias creeping up in AI recruitment tools; for example, Amazon’s algorithm became biased towards women. Even after gender pronouns and first names were removed from resumes, the tool still favoured men by considering certain keywords traditionally associated with men. How can something like this be corrected?
MB: Unfortunately the results were based on data derived from how our society has lived in the past century and there is an important lesson which must be learnt from this blunder.
AD: Its easier to blame AI, but the culprit is the bad data that we are feeding to AI, which is completely our fault. We must be able to quantify the biases and eliminate them before using such data for deep learning.
DV: Nema dileme, nisu krive ni mašine ni podaci, već ljudi koji su programirali softver. Ponovo se vraćam na ulogu i značaj domenskih eksperata. Na žalost, nedovjlno se poštuje uloga ljudskih eksperata koji MORAJU imati svoje mestu u AI (data science) timu. Programeri, matematičari, statističari, menadžeri i ostali članovi tima ne mogu da znaju prirodu problema koji AI treba da rešava, preuzimajući nekritički podatke iz nekakvih baza podataka. Čovek – ekspert za određenu oblast, mora da ugrađuje sopstveno znanje u svim fazama životnog ciklusa procesa “opamećivanja” mašina. Već smo odgovarali na pitanje vezano za iskrivljenost u podacima. Ona je i ovde prisutna, u kategoriji koje se uobičajeno ne smatra „marginalizovanim“, već „gender issue(s)“, sa devijacijama koje su više nego očigledne.
Pri tome MORA da se poštuje poznata naučna metodologija koja nedvosmisleno definiše svaku fazu u implementacijii, ako već ne postoji jedna, jedinstvena metodologija na kojoj se baziraju AI rešenja.
But how can we correct these biases? Do we keep collecting more data which still may not be representative of the whole population? What is the most accurate data to learn from eventually?
AD: Google has already started the conversation on this and is laying down principles to govern the whole idea of data sharing by linking data trust to the notion of ownership and leveraging from today’s age of social media. But we still don’t know if we will be able to achieve this utopic vision because not everyone shares data on social media, so it’s hard to have a balance.
DV: Greška je napravljena već u postavci pitanja… u kome se podaci apostrofiraju za glavnog krivca za pristrasnost. Moj odgovor je sadržan u prethodnim komentarima – krivica leži u ljudima koji treba da upravljaju mašinama (a ne obrnuto).
The enlightened audience had several thought-provoking questions as well.
Q: How do we educate people about AI ethics and engage them regarding its applications when they don’t even understand what AI actually is and most people think of it as a real-life terminator?
A: Scientists need to get out of their cocoons and start discussing their research with a wider audience so that they can obtain adequate feedback before developing further applications. It’s necessary to engage communities and present talks to be able to get a real-world view of what this technology is capable of and what kind of impact it can have on society. We must bridge the gap between us and make the community more familiar with AI.
DV: Među samim naučnicima postoji duboki jaz i podela. Usled nasleđenih ili stečenih sujeta, oni ne prihvataju suočenje sa realnošću: praksa nema vremena da čeka njihove teorije. I zato se javljaju teze poput: Big Data (slično važi i za AI) predstavlja praksu bez teorije („The no theory thesis”), dok neki autori čak idu do toga da navode očekivanje i/ili strahovanje da Big Data predstavlja kraj teorije u nauci (Leonelli, 2014; Mazzocchi, 2015) Big Data. Onda i ne čudi odakle dolazi pristrasnot. Neproduktivno je naknadno likovanje akademske javnosti i ukazivanje na sopstvenu neophodnost, pre bi moglo da se govori o sopstvenoj ODGOVORNOSTI. Takođe, naučnici moraju što pre da shvate da moraju što pre da izađju iz svojih kabineta i amfiteatara i suoče sa drugim kolegama i PRAKSOM.
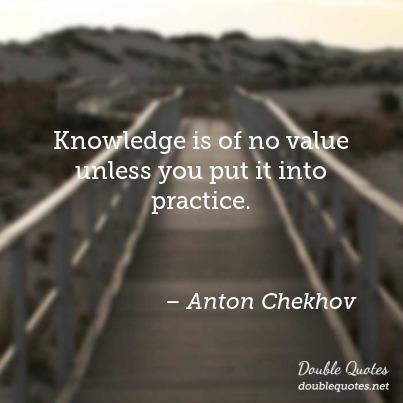
Interdisciplinarni pristup isključuje nasleđe: jedan naučnik – jedna disciplina (medicina nas je dosta naučila o tome – holistički pristup). Obrazovni proces je posebna priča. Ko će školovati ljude koji će školovati mašine? I kako? Sigurno, ne kao do sada.
Q: You said human intervention is essential to regulate AI so that it doesn’t develop any biases. But the human beings carrying out that job may still not be representative of the whole population. Then how can you ensure that AI will be fair for all?
A: That’s almost impossible to achieve, but more research needs to be done to find a solution.
DV: sve dok su ljudi pristrasni, biće i mašine. Jedina razlika je u tome što možemo računati na doslednost AI (sa istim inputima mašina daje iste outpute) – (što ne krasi ljudsku populaciju, naprotiv) – mašina neće menjati svoje mišljenje dok ih ne naučimo drugačije.
„Therefore, the degree of uncertainty in AI made decisions is lower than that made by human beings. For example, if one asks human beings to make decisions at different times they will change their minds depending on all sorts of irrelevant factors“. (Marwala & Hurwitz)
Ponoviću, buduće napore treba usmeriti ka razvijanju standarda koji važe, pre svega među ljudima. Ovo se ne može postići na globalnom nivou. Npr. Ne očekujem da će se stav prema određenim grupama izjednačiti širom sveta, Rusiji, npr. Pada mi na pamet, kao jedno od rešenja uvođenje standarda sličnih onima koji se koriste u zvaničnoj statistici – meta podaci, standardni izveštaji o kvalitetu itd.
Q: Do you suggest any strategies or frameworks to incorporate the concept of non-binary genders into AI research and development?
A: This again is a niche subject which needs to be explored further and unless we have data that is representative of all human beings, irrespective of their backgrounds, ethnicities, genders or sexual orientations, it’s difficult to suggest any strategies, yet significant work is being done in this regard.
DV: Vratiću se na nivo statističkih standarda, shodno prethodno pomenutom odgovoru. Koncept “Gender identity” nije baš ni tako nov. Još u decembru 2007, Vrhovni sud u Nepalu objavio je revolucionarnu presudu u korist seksualnih i rodnih manjina. Odluka u predmetu “Pant protiv Nepala (Pant v. Nepal)” brzo je postala široko poznata po proglašenju punih, osnovnih ljudskih prava za sve „Seksualne i rodne manjine“ – lezbijska, gej, biseksualna, transrodna i interseks (LGBTI) građanke i građane.
Naravno, potrebno je poći od krovne legislative koja treba da definiše rodni identitet, rodni izraz ili polne karakteristike, a recimo i to da ovakva legislativa na nivou EU ne postoji:
“EU primary legislation contains no explicit references to gender identity, gender expression or sex characteristics, although the Charter does list sexual orientation in the list of non-discrimination grounds (Art. 21(1))”.
Jedno od rešenja je da se definiše standard za uvođenje non-binary individuals (gender) kategorije. Svakako, kategorija “Pol pri rođenju” može dodatno da se uvede.
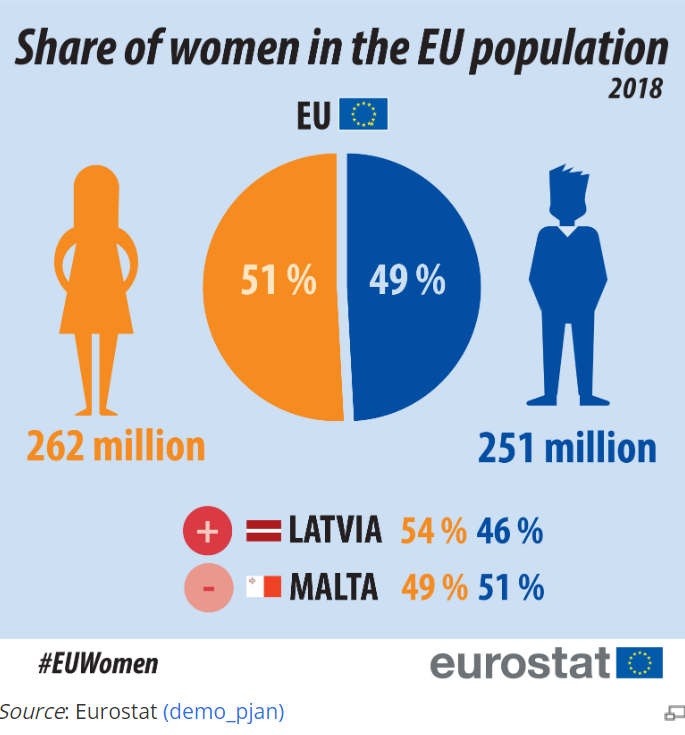
The panel was invigorated by such challenging and stimulating questions and probably went home with some good inputs on how the concept of fairness must be incorporated into AI, so that it can champion equality without any discrimination or biases.
This elucidative evening ended with a befitting comment from one of the audience members who claimed to be the oldest among a predominant group of millennials:
“Let AI mature for the future with further research and development, but for now, let us primarily focus on its current applications and how they can be utilized to have a more just and inclusive society in the present day world.”
Queer Tech Montreal has over a thousand members and we want you to be a member of our community group for LGBTQ+ professionals in the tech and start-up sectors. All are welcome.
Keep in touch and be a member of our Meetup group, and follow us on Facebook and Twitter.